Databases of health data are widely shared among researchers and for commercial purposes, and they are even put online in order to promote health research and data-driven health app development, so preserving the privacy of patients is critical. But are these data sets de-identified properly? If not, it could be re-identified. Just look at the two high profile re-identification attacks that have been publicized in recent months.
The first attack involved individuals who voluntarily published their genomic data online as a way to support open data for research. Besides their genomic data, they posted their basic demographics such as date of birth and zip code. The demographic data, not their genomic data, was used to re-identify a subset of the individuals.
The second attack used hospital discharge data from Washington State—this data was available for a small fee. The discharge database contained information about the date of the admission, the patient zip code, and the diagnosis. The attackers used the following techniques to re-identify individuals:
-
They matched records against newspaper articles about incidents such as road accidents, which gave names of individuals, the date, the hospital that the individual was taken to, and descriptions of the incidents.
-
They paired the name of the individual from the article with the individual’s ZIP code from the white pages.
-
Knowing the ZIP code, date, hospital, gender, and diagnosis made it relatively straight forward to find the individual’s record in the hospital discharge database.
These techniques of matching basic demographics with publicly available information have been known since the 1990s.
The health care field is not learning from its mistakes. Washington State was still disclosing hospital discharge data with no meaningful de-identification practices or controls. Individuals continue to post sensitive health information with their dates of birth and zip codes, believing mistakenly that it is anonymous just because their names are not included.
Today, we have some comprehensive guidelines and standards for the de-identification of health information. Unfortunately, these have not been fully disseminated to those who can make the best use of them, as is clearly demonstrated by the above two examples.
As we cannot revert to the way things used to be and stop acquiring and sharing data, we must now ensure that data is collected and shared responsibly. Adopting de-identification standards is absolutely necessary if we want to protect our sensitive data, identity and privacy. But it is also necessary if we want to avoid a negative regulatory or public reaction that would curtail access to health data. This would limit the substantial societal and commercial benefits of such access.
De-identification standards are part of the privacy-by-design toolbox. De-identification standards cannot be implemented in a vacuum – they need to be implemented with an organizational context with policies, templates, training, and well defined de-identification services for different use cases. To achieve these objectives, organizations can implement the De-identification Maturity Model (DMM), developed by Privacy Analytics, which is a framework for evaluating the maturity of de-identification services within an organization. The framework gauges the level of an organization’s readiness and experience regarding de-identification of people, processes, technologies and consistent measurement practices. Implementing the DMM provides organizations with a measurement tool that evaluates their de-identification practices, provides a roadmap for improvement, and helps them determine what is required to improve their de-identification practices.
As a recent example, we used the DMM to assess the practices of a large health department. This highlighted the variability that existed within the department with respect to how data is shared and de-identified. Specific best practices were identified that could be more rapidly shared across the department, and a general roadmap was developed to allow the various divisions to move towards the implementation of best practices, and have these supported by the enterprise. The expected outcomes are faster data releases, and more defensible protection of patient privacy. Another critical “soft” outcome was the ability to generate buy-in within the organization to implement these best practices because they would allow them to be better able at meeting their business mandates (i.e., the services they are expected to provide).
To answer the question posed in the title: Yes, we do have to worry. Attacks like the ones described will become more common. Researchers and the media will continue to illustrate how poorly de-identified data can be attacked. There is even now a “code of conduct” for re-identification professionals explaining how to conduct re-identification attacks responsibly: Breaking Good: A Short Ethical Manifesto for the Privacy Researcher. Re-identification is becoming a discipline. Data custodians need to implement responsible practices that will allow them to manage re-identification risks while still benefiting from data collection, sharing and analytics. Regulators can also play an important role in developing and disseminating de-identification standards.
Implementing responsible practices and developing and disseminating de-identification standards, as I do in the book Anonymizing Health Data and in my upcoming Strata Rx session, will help organizations and individuals repel re-identification attacks and avoid situations where re-identification is easily achieved.
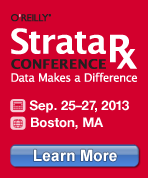
Strata Rx Heath Data Conference — Strata Rx brings together the diverse communities driving innovations in big data analytics for health care. Learn about the transformation of health care through big data and how to position your company to benefit from these trends. Learn more.